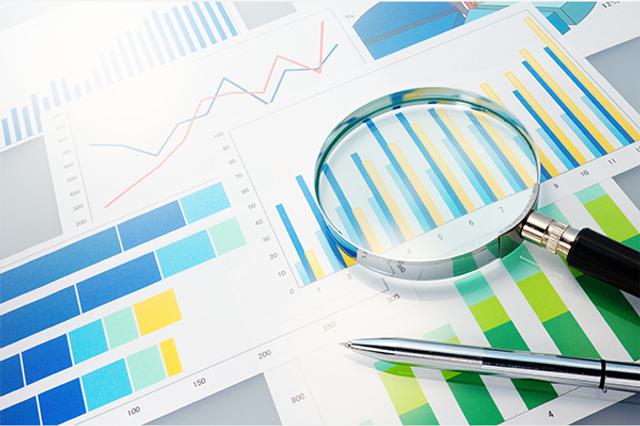
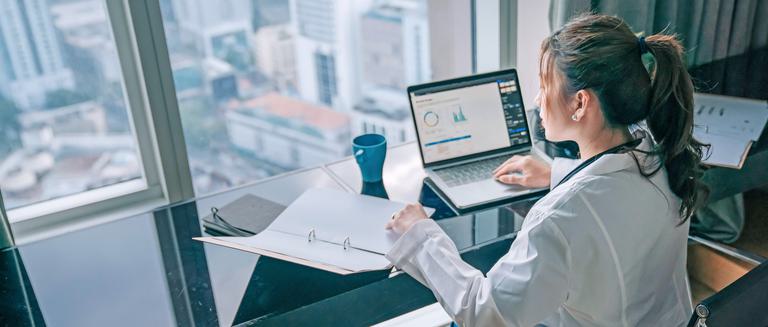
Custom MLOps Platform Improves Performance for a Leading Health Insurer
CapTech was engaged to oversee the transition, including the migration of science models and related artifacts and the integration of a custom-built MLOps.
Summary
A leading health insurer wanted to optimize its healthcare analytics operations and infrastructure to automate tasks, improve efficiency, and open the door for future innovation. The long-term goal was to transition from an on-premises Cloudera environment to a more contemporary Google Cloud Platform (GCP). CapTech was engaged to oversee the transition, including the migration of state-of-the-art data science models and related artifacts and the integration of a custom-built Machine Learning Operations (MLOps) platform on Vertex AI within the new GCP environment.
Challenge
The insurer’s data science team was being weighed down by operational tasks and overseeing manual processes, preventing them from focusing on finding new ways to drive innovation within the organization. The team was encountering prolonged delays in monthly scoring analytics, and the looming prospect of cloud migration further complicated matters. Data availability issues were prominent, creating delays in model completion and increasing stress due to impending deadlines.
The process of model creation was hindered by a lack of clarity into the data features behind the custom logic, which had difficulty accommodating new features. One significant inefficiency was the amount of time research scientists spent on repetitive manual tasks like cohort creation, manual pipeline triggering, and general platform maintenance. Additionally, the Cloudera cluster presented its own set of challenges - from the need for manual coordination to schedule runs to consequent hardware and computational bottlenecks. The restrictive nature of certain tools, which were exclusive to the Cloudera ecosystem, further constrained their operations.
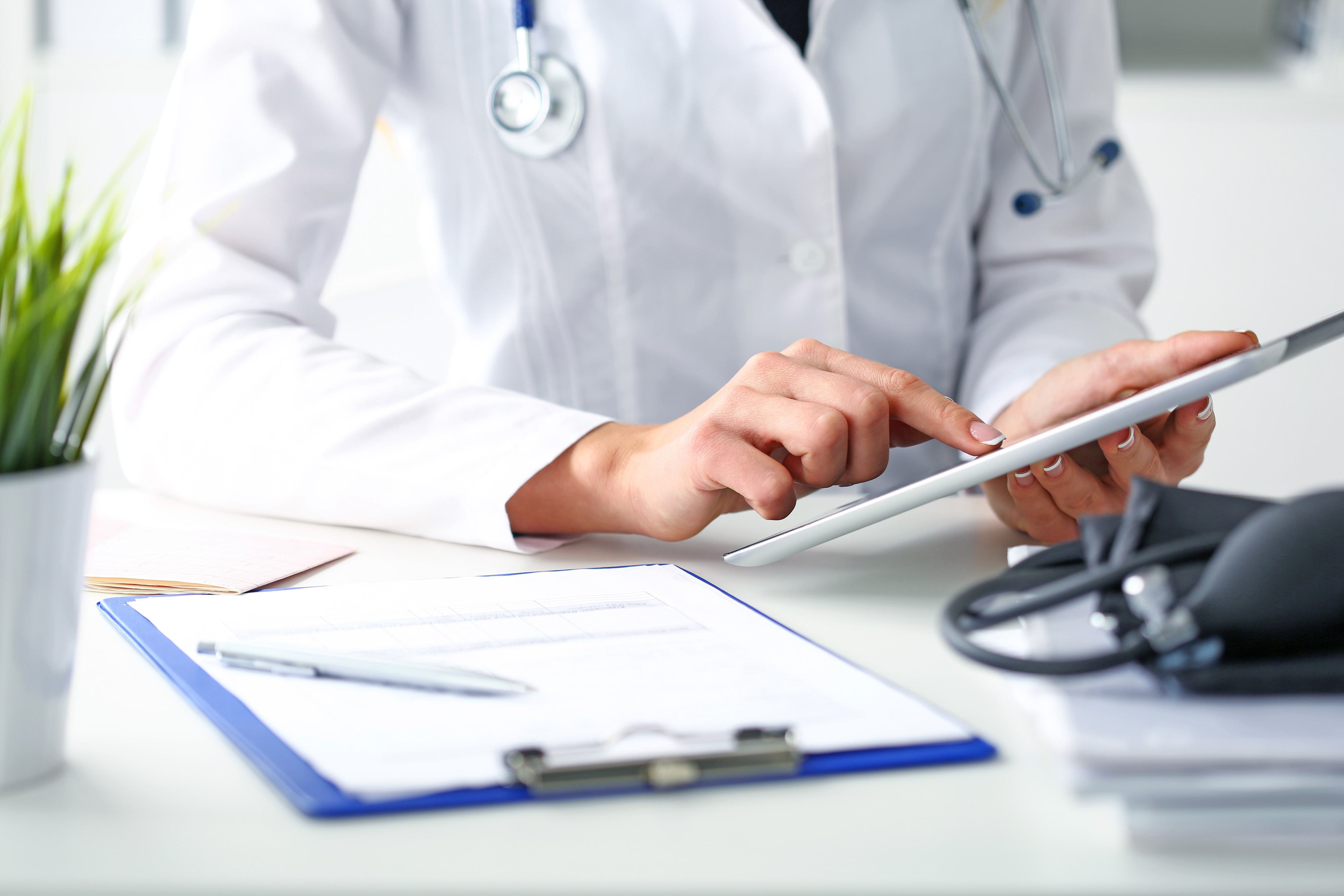
Approach
To remedy these challenges, CapTech collaborated with the client to conduct a comprehensive architectural and cloud migration assessment, resulting in a set of actionable recommendations. With these insights, CapTech then migrated all of the insurer’s data science models from Cloudera to GCP. Subsequent steps involved developing a tailored MLOps framework and process that would be used by the team of analysts, data scientists, and engineers. This new platform was intended to automate model runs, minimize errors, boost performance, and reduce infrastructure costs. With the foundation in place, there was a consistent drive to develop new models while ensuring the existing ones were well-maintained in the new analytics environment.
Selected Models Impacted/Developed:
- Likelihood of Readmission
- Likelihood of Hospitalization
- Risk of Diabetes Onset
- High-Risk Pregnancy Identification
- Predicted High Cost
- Customer Service Call Reason (NLP)
- Customer Service Call Quality (NLP)
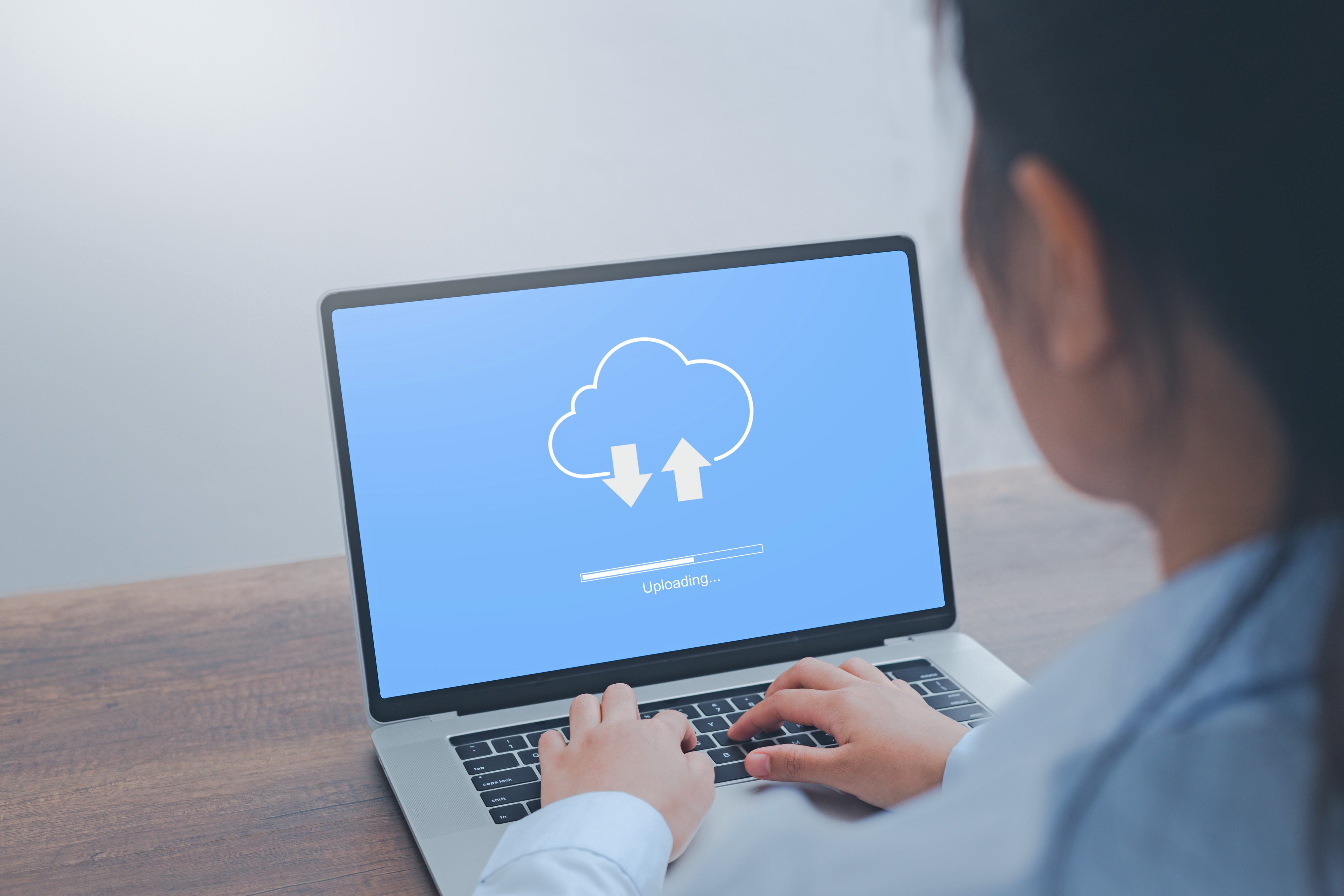
Additionally, CapTech took a lead role in developing the first predictive model fully integrated into the new platform. This model focuses on predicting shifts of members from "normal" to "high" cost categories in the coming year. In addition to these predictions, the solution provides valuable insight by offering an explainability feature. This feature sheds light on the specific clinical events influencing the model's decisions, fostering a transparent and informed approach to healthcare decision-making.
Results
- Reduction in delivery time
- 23%
The transformation project:
- Reduced delivery time frame, from data availability in Teradata to its presentation to stakeholders, from 10-15 days to just 3-5 days in the GCP environment.
- Improved the performance of all models due to the auto-scaling infrastructure built by the CapTech team.
- Improved human resources allocation by automating model operations and eliminating the multi-day downtime that was previously needed to run the process manually each month.
- Reduced infrastructure costs by 30% month-over-month.